Understanding the Impact of AI in Outbound Calling
In today’s sales environment, artificial intelligence has transformed how businesses conduct outbound calls. AI-powered systems can now analyze customer data, predict responses, and even conduct entire conversations with prospects. However, many organizations fall into common traps when implementing these solutions.
The integration of AI in sales isn’t just a technological upgrade—it represents a fundamental shift in customer engagement strategy. According to recent research by Salesken.ai, companies using AI for outbound calling report up to 300% increase in connection rates compared to traditional methods.
But success with AI calling isn’t automatic. Many businesses invest heavily in AI technology without understanding the nuances required for effective implementation. This leads to wasted resources and potentially damaged customer relationships.
The most common misconception is treating AI as a complete replacement for human representatives rather than as a powerful augmentation tool. As we’ll explore, finding the right balance between automation and personalization remains the key challenge for businesses implementing AI outbound calling solutions.
Neglecting Proper AI Training and Data Preparation
One of the most devastating mistakes organizations make is deploying AI calling systems without adequate training or data preparation. Your AI is only as good as the data it learns from, and skipping this crucial foundation leads to poor performance.
High-quality training requires diverse conversation samples that represent various customer scenarios, objections, and responses. Many companies rush this process, feeding their AI systems with limited datasets that fail to capture the complexity of real customer interactions.
Beyond conversation samples, AI for sales needs accurate customer data to personalize outreach effectively. When your AI works with outdated or incorrect information, it creates jarring experiences that immediately reveal its non-human nature.
According to Fireflies.ai, companies should dedicate at least 3-4 months to properly train their AI systems before full deployment. This investment pays dividends through significantly improved performance and customer experience.
Failing to Establish Clear Disclosure Protocols
Transparency about AI involvement in calls isn’t just an ethical consideration—it’s increasingly becoming a legal requirement in many jurisdictions. Failing to properly disclose when customers are speaking with an AI system can severely damage trust and potentially expose your business to regulatory penalties.
The disclosure dilemma presents a challenging balance: how to be transparent without immediately triggering negative reactions from customers who might hang up upon learning they’re speaking with AI. The key lies in framing the AI as a tool to provide better service rather than a cost-cutting measure.
Best practices include a brief, clear disclosure at the beginning of the call that avoids technical jargon. For example: "Hi, I’m Jake from ABC Company. I’m an AI assistant calling to discuss our new service offering." This approach is preferred over vague statements that might mislead customers into believing they’re speaking with a human.
Studies from Reddit discussions on AI calling show that customers respond more positively when disclosure is handled confidently and matter-of-factly rather than apologetically. Remember that conversational AI continues to improve, and many customers are increasingly comfortable with these interactions when they’re handled transparently.
Over-Automating the Human Connection
The greatest strength of human salespeople—building genuine rapport and emotional connections—remains the biggest challenge for AI calling agents. Companies frequently make the mistake of automating too much of the conversation, creating robotic interactions that fail to resonate with prospects.
Ironically, the most successful AI calling implementations maintain a delicate balance between automation and personalization. While AI can handle scheduling, information gathering, and basic objection handling, complex emotional signals still require human intervention.
Some organizations attempt to overcome this by programming their AI with overly casual language or slang in an attempt to sound more human. This approach typically backfires, creating an "uncanny valley" effect where the AI seems almost human but slightly off in ways that make customers uncomfortable.
A better approach, as demonstrated by platforms like Callin.io, is to embrace the AI’s strengths in consistency and information processing while designing thoughtful escalation points for human involvement. For example, using AI for initial outreach and qualification, then transitioning promising conversations to human sales representatives for relationship building.
Ignoring Compliance and Legal Requirements
The regulatory landscape for AI calling continues to evolve rapidly, and failing to stay current with regulations can result in significant penalties. Many businesses mistakenly assume that traditional telemarketing laws don’t apply to AI systems or that existing compliance measures are sufficient.
Different regions have varying requirements regarding call recording notifications, consent for automated calls, and specific restrictions on when calls can be placed. The Telephone Consumer Protection Act (TCPA) in the United States, GDPR in Europe, and similar regulations globally all have implications for AI calling programs.
Beyond explicit telemarketing laws, businesses must consider data protection regulations governing how customer information is collected, stored, and utilized by AI systems. This becomes particularly complex when training AI on real customer conversations, which may contain sensitive information.
Research from Hyperbound.ai indicates that companies implementing comprehensive compliance programs for their AI calling initiatives experience 40% fewer customer complaints and significantly reduced legal risks. Working with legal counsel familiar with both telemarketing regulations and AI applications is essential before launching any outbound AI calling program.
Setting Unrealistic Performance Expectations
Many organizations implement AI calling with wildly unrealistic expectations about its capabilities and performance, leading to disappointment and premature abandonment of potentially valuable programs. The mistake lies in expecting AI to immediately outperform human agents across all metrics.
While AI excels in consistency, availability, and processing large volumes of calls, it still faces limitations in handling unexpected scenarios, complex objections, or emotionally charged conversations. Companies frequently overlook these limitations during planning stages, setting targets that even the most advanced AI systems can’t currently achieve.
According to Insight7.io, businesses should expect their first AI implementation to match average human performance in specific, well-defined scenarios—not to replace their top salespeople. Success metrics should focus on consistency, compliance, and specific use cases where AI demonstrates measurable advantages.
The most successful implementations start with narrow, clearly defined use cases where AI can excel—such as appointment setting or qualification calls—before gradually expanding to more complex scenarios. This measured approach allows both the technology and the organization to evolve together, building on successes rather than becoming discouraged by early limitations.
Choosing Inflexible AI Calling Solutions
When selecting an AI calling solution, many businesses prioritize current features over adaptability and future development potential. This shortsighted approach quickly leads to disappointment as business needs evolve or new capabilities become available.
The AI calling landscape is advancing rapidly, with new capabilities emerging monthly. Organizations locked into rigid platforms find themselves unable to incorporate these innovations without disruptive and expensive system migrations. The true value of an AI calling platform lies not just in its current capabilities but in its ability to evolve alongside your business.
Key flexibility considerations include API access for custom integrations, the ability to incorporate proprietary data and training materials, and regular feature updates from the provider. Solutions like Bland.ai and others highlighted in recent YouTube comparisons demonstrate significant differences in how platforms approach ongoing development.
Industry experts recommend evaluating potential providers based on their development roadmap, update frequency over the past year, and flexibility in accommodating customer-specific requirements. The most successful implementations typically come from providers who view their relationship with customers as a partnership in ongoing development rather than a one-time software purchase.
Neglecting Integration with Existing Systems
A common and costly mistake is implementing AI calling as a standalone solution without proper integration into existing CRM, sales, and customer service systems. This creates information silos that severely limit the AI’s effectiveness and creates frustrating experiences for both customers and internal teams.
Effective AI calling requires seamless data flow between systems to provide agents with context-rich information during calls and to capture valuable insights for future interactions. When integration is overlooked, sales teams must manually transfer information between systems, negating much of the efficiency AI promises.
Beyond basic data sharing, robust integrations enable powerful workflows where AI calling triggers appropriate follow-up actions. For example, sending personalized emails after calls, scheduling follow-up appointments in sales representatives’ calendars, or updating opportunity stages in your CRM system.
Research from Sales-mind.ai shows that organizations with tightly integrated AI calling systems achieve 65% higher conversion rates compared to those using standalone solutions. When evaluating AI calling platforms, prioritize those with pre-built integrations for your existing technology stack or robust API capabilities for custom integration development.
Lack of Human Oversight and Quality Control
While the promise of fully autonomous calling is attractive, businesses that eliminate human oversight entirely experience serious quality issues and missed improvement opportunities. AI systems require ongoing monitoring and guidance, particularly in their early implementation stages.
Effective oversight includes regularly reviewing call recordings, analyzing performance metrics, and providing feedback to refine AI behaviors. Companies that implement structured review processes identify patterns in customer reactions and objections that can be addressed through targeted AI training.
Beyond quality control, human oversight provides crucial risk management by identifying potential compliance issues, brand misalignment, or scenarios where the AI struggles to provide appropriate responses. This feedback loop accelerates the AI’s learning curve and prevents small issues from becoming significant problems.
According to experts at Air.ai, the ideal oversight model evolves over time, with more intensive human involvement during initial deployment gradually transitioning to exception-based reviews as the AI demonstrates consistent performance. However, even the most advanced systems benefit from ongoing human guidance to adapt to changing market conditions and customer expectations.
Failing to Prepare for the Handoff to Human Agents
Many organizations implement AI calling without a clear strategy for transitioning promising conversations to human sales representatives. This critical handoff moment can quickly undermine all the benefits of AI outreach if handled poorly.
The most common mistake is creating a disjointed experience where human agents lack context about the preceding AI conversation. This forces customers to repeat information and creates the impression of organizational disconnection. Effective handoffs require both technical integration and procedural clarity.
On the technical side, human agents need immediate access to conversation transcripts, key points discussed, and any commitments made by the AI. Platforms like Evecalls specialize in creating seamless transitions where human agents can quickly absorb context before engaging.
Procedurally, organizations need clear criteria for when handoffs should occur and training for human agents on how to acknowledge and build upon the AI interaction rather than treating it as separate. The language used during these transitions significantly impacts customer perception of both the AI and the organization as a whole.
Using Generic Scripts and Responses
One of the most visible mistakes in AI calling is relying on generic, one-size-fits-all scripts that fail to address the specific needs and characteristics of different customer segments. This approach immediately signals to customers that they’re receiving standardized treatment rather than personalized attention.
Effective AI calling requires multiple script variations tailored to different customer personas, industries, and scenarios. These scripts should incorporate relevant terminology, address industry-specific challenges, and reference appropriate use cases that resonate with each segment.
Beyond initial segmentation, AI for resellers and other specialized applications require unique approaches that acknowledge the specific dynamics of these relationships. The most sophisticated implementations dynamically adjust language based on customer responses, creating truly adaptive conversations.
Research published on Callin.io’s blog demonstrates that AI systems using segment-specific scripts achieve 83% higher engagement rates compared to those using generic approaches. While developing these variations requires additional upfront investment, the return in terms of call effectiveness and conversion rates makes this one of the highest-impact improvements for AI calling programs.
Overlooking Voice Quality and Naturalness
The technical quality of AI voices significantly impacts customer perception, yet many organizations implement systems with robotic or unnatural-sounding voices that immediately signal an automated interaction. This undermines trust and engagement before the conversation even begins.
Recent advancements in text-to-speech technology have made highly natural AI voices available, but implementing them effectively requires attention to several factors beyond base technology. These include appropriate pacing, natural pauses, emotional inflection, and pronunciation of industry-specific terminology.
Leading providers like ElevenLabs and Play.ht offer voices optimized for conversation rather than simple narration, with capabilities for contextual emphasis and emotional variation. Regional considerations are also important, with options like the German AI voice for specific market requirements.
A common misconception is that more human-sounding voices should always be preferred. However, research from Cartesia AI suggests that slightly recognizable AI voices often perform better than those attempting perfect human mimicry, as they set appropriate expectations for the interaction while still delivering a pleasant experience.
Lack of Continuous Improvement Processes
Implementing AI calling as a "set it and forget it" solution guarantees diminishing returns over time. Without structured improvement processes, initial performance gains quickly plateau as market conditions change and customer expectations evolve.
Effective continuous improvement requires systematic collection and analysis of performance data, including conversion rates, objection patterns, call duration metrics, and customer feedback. This analysis should drive regular updates to scripts, responses, and conversation flows.
Many organizations fail to establish clear ownership for ongoing AI optimization, leaving these systems to gradually deteriorate without the focused attention needed for sustained performance. Successful implementations typically assign specific responsibility for AI performance to a role or team with appropriate metrics and incentives.
The most sophisticated approaches implement A/B testing methodologies, simultaneously running different versions of scripts or approaches to identify improvements through direct comparison. Platforms that support this kind of structured experimentation, like those discussed in how to break into AI sales, deliver significantly better long-term results compared to static implementations.
Insufficient Preparation for Handling Objections
Even the most advanced AI calling systems struggle when confronted with unexpected objections or complex questions. Organizations frequently underestimate the variety of objections their AI will encounter, programming responses for only the most common scenarios.
This preparation gap creates awkward moments where the AI either fails to recognize the objection or provides generalized responses that fail to address customer concerns. The resulting impression is of a system that listens poorly and lacks real understanding—precisely the opposite of what effective sales conversations require.
Comprehensive objection handling requires cataloging actual customer responses from previous calls, developing specific responses for each objection type, and creating graceful fallback options for situations beyond the AI’s capabilities. The most effective systems combine specific responses for common objections with classification capabilities that can identify novel objections requiring human intervention.
Training materials from Hyperbound.ai recommend developing at least 15-20 objection response variations for each major objection category, allowing the AI to select contextually appropriate responses rather than repeating identical language across calls. This diversity significantly improves perception of the AI’s conversational capabilities.
Disregarding Cultural and Regional Considerations
International businesses often make the critical mistake of applying uniform AI calling approaches across different regions without accounting for cultural norms, communication preferences, and regulatory differences. This one-size-fits-all approach creates particularly poor experiences in markets where business communication norms differ significantly from the organization’s home region.
Beyond obvious considerations like language and time zones, effective regional adaptation includes adjusting conversation pacing, formality levels, and even the basic approach to building business relationships. In some cultures, direct discussion of business opportunities without relationship building is considered inappropriate, while in others, extended pleasantries may be viewed as wasting time.
Regulatory requirements also vary significantly by region. The Twilio BYOC guide highlights how telecommunications compliance differs across regions, with implications for AI calling implementations regarding consent requirements, recording disclosures, and data handling.
Organizations successfully navigating these challenges typically create region-specific AI calling programs with dedicated scripts, voices, and compliance measures rather than attempting to adapt a single approach globally. While this increases implementation complexity, the improved customer experience and reduced compliance risks justify the investment.
Ignoring Emotional Intelligence in AI Design
Perhaps the most fundamental mistake in AI calling implementation is treating these systems as purely transactional tools rather than conversational agents requiring emotional intelligence. The most technically sophisticated AI will fail if it cannot recognize and appropriately respond to emotional cues from customers.
Emotional intelligence in AI calling includes recognizing signs of customer frustration, confusion, or interest, and adapting conversation flow accordingly. This capability goes beyond simple sentiment analysis to include appropriate responses that acknowledge and address emotional states rather than proceeding with scripted sequences regardless of customer reaction.
Implementing emotional intelligence requires training AI to recognize vocal cues like tone, pace, and volume changes that signal emotional states. It also involves programming appropriate responses to diffuse negative emotions or capitalize on positive engagement. The AI phone consultant guide explores how these capabilities are evolving through advanced natural language processing.
While perfect emotional intelligence remains beyond current AI capabilities, even basic implementation significantly improves customer experience. Simple measures like programmed pauses after customer statements, acknowledgment phrases for emotional cues, and escalation paths for detected frustration can dramatically improve how customers perceive AI interactions.
Elevate Your Outbound Calling Strategy with AI-Powered Solutions
After exploring the common pitfalls in AI outbound calling, it’s clear that successful implementation requires thoughtful strategy, ongoing refinement, and the right technology partner. The difference between disappointing results and transformative outcomes often comes down to how thoroughly these mistakes are addressed.
Implementing AI calling isn’t simply about adopting new technology—it’s about reimagining customer conversations for the digital age. Organizations that approach this transformation with careful planning, realistic expectations, and commitment to continuous improvement can achieve remarkable results in efficiency, consistency, and customer experience.
If you’re looking to transform your business communications with intelligent, automated solutions, Callin.io offers a comprehensive platform for implementing AI phone agents that handle both inbound and outbound calls autonomously. Their innovative technology enables you to automate appointment scheduling, answer frequently asked questions, and even close sales through natural customer interactions.
Callin.io’s free account provides an intuitive interface for configuring your AI agent, with test calls included and access to a task dashboard for monitoring interactions. For businesses requiring advanced capabilities like Google Calendar integration and built-in CRM functionality, subscription plans start at just $30 per month. Discover how Callin.io can help you avoid these common mistakes and implement truly effective AI calling solutions by visiting their website today.
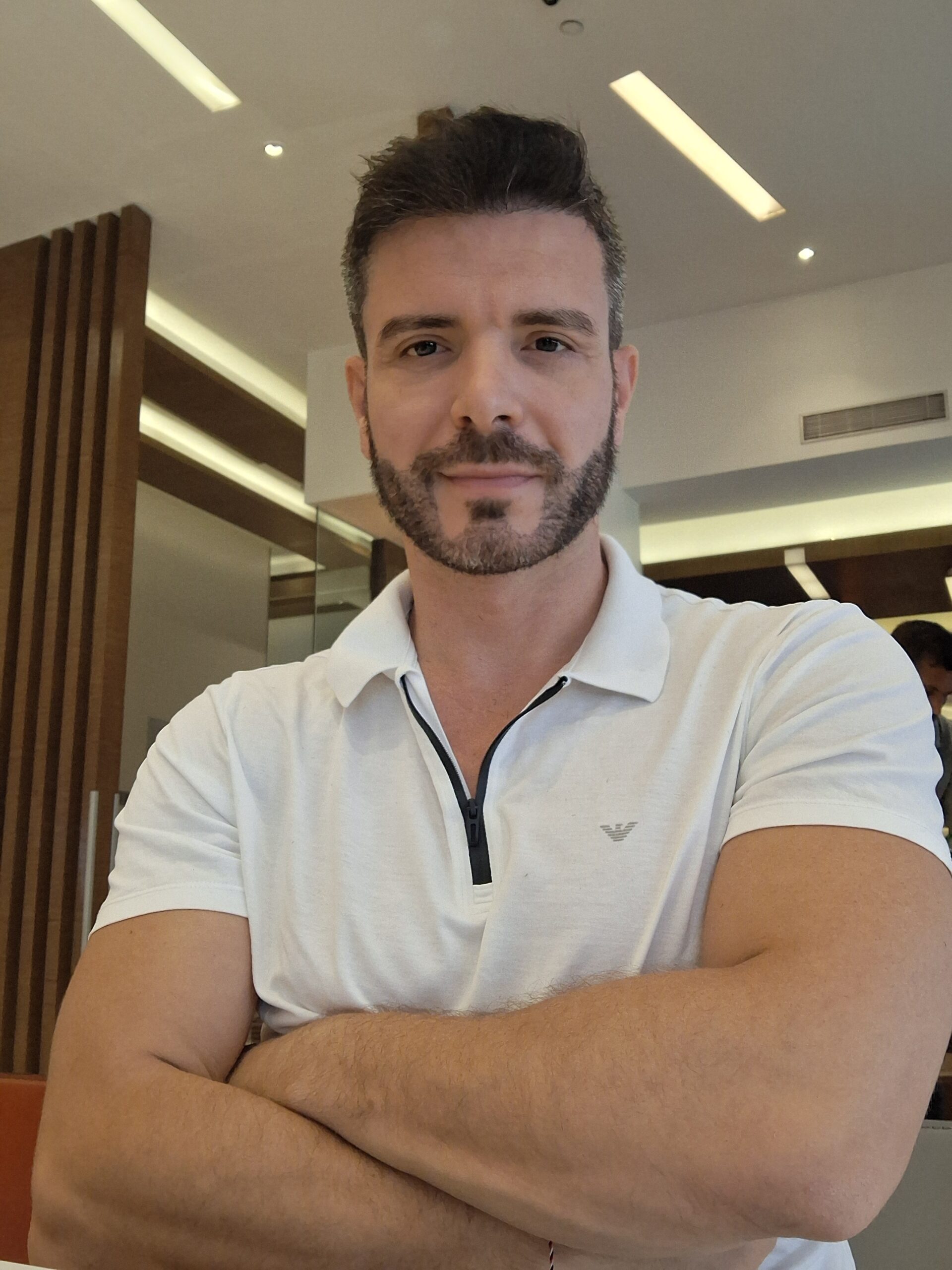
Helping businesses grow faster with AI. 🚀 At Callin.io, we make it easy for companies close more deals, engage customers more effectively, and scale their growth with smart AI voice assistants. Ready to transform your business with AI? 📅 Let’s talk!
Vincenzo Piccolo
Chief Executive Officer and Co Founder